5 Tips for Faster Troubleshooting to Reduce MTTR
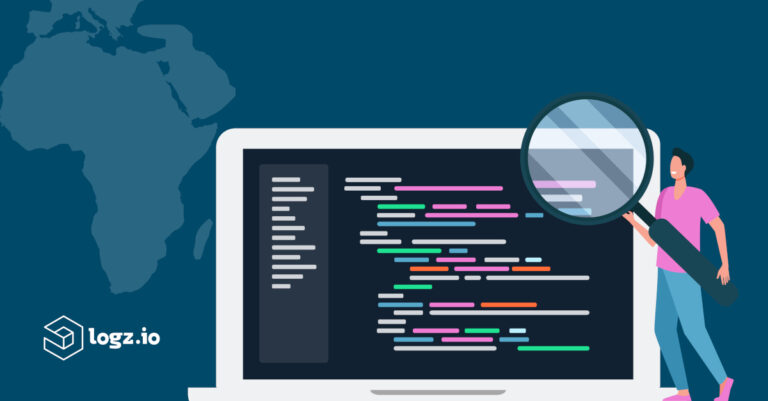
In today’s rapidly evolving digital landscape, organizations heavily rely on their applications and systems to deliver optimal performance. As such, driving down the key metric of Mean Time to Resolution (MTTR) is clearly one of the biggest challenges facing observability practitioners today.
According to the 2024 Observability Pulse Report, based on our annual survey of global IT and DevOps leaders, over 80% of respondents said their current pace of MTTR exceeds multiple hours, continuing a trend of the past several years. As a result, only 9% of respondents stated they were satisfied with their current MTTR, indicating an urgent need for improvement.
Today, we’ll explore five essential tips for faster troubleshooting to reduce MTTR and ensure optimal performance using some proven observability practices. By implementing these strategies, organizations can streamline their troubleshooting processes, minimize downtime, and deliver exceptional user experiences.
Tip 1
Automated Data Insights
Automation plays a vital role in accelerating troubleshooting processes. By leveraging artificial intelligence (AI) capabilities, organizations can automate log analysis and gain valuable insights quickly. Tools that cluster logs into patterns and highlight critical exceptions help teams focus on the most relevant information and reduce manual search efforts.
Systems that integrate generative AI and sentiment analysis can assist in troubleshooting by providing recommended remediation actions and surfacing critical log data. These automation features enable faster log search and analysis, reducing the time spent on identifying and resolving issues. Natural language search, enabled by LLM integration, empowers users to move from complex querying to having a direct conversation with their data.
By harnessing the power of automated data insights, organizations can improve MTTR, optimize resource utilization, and enhance overall system performance.
Tip 2
Root Cause Analysis
Another way that automation can play a factor in troubleshooting issues faster is through AI-powered root cause analysis (RCA). Teams can carry out investigation faster with RCA, notably eliminating numerous manual investigation steps, removing the need to pivot between multiple dashboards, enact numerous queries or filter through a vast number of events to carry out in-depth troubleshooting. There’s an instant understanding of where a specific problem was introduced, including recent deployments.
Detailed insights can be generated into any alerts, while automatically generating related conclusions and response steps, reducing MTTR. Pinpointing the causes and implications of existing alerts will identify the most informed, efficient, and timely resolution actions that mitigate impacts.
Tip 3
Correlating and De-Risking Deployments
In today’s fast-paced software development environment, frequent deployments and code changes are common. Understanding the ongoing impact of these changes on system performance is crucial for efficient troubleshooting.
By overlaying deployment information on telemetry data, organizations can quickly identify correlations between deployments and issues. This allows teams to determine if a recent deployment is causing performance degradation or errors, enabling them to take appropriate actions, such as rolling back the changes in question.
By using AI-powered analysis, you’ll immediately understand the impact of newly or recently deployed code and configurations, dramatically lowering the risk of CI/CD practices. By closely tracking and analyzing changes, organizations can ensure smoother deployments, minimize system disruptions, and optimize overall system stability.
Tip 4
Application Performance Investigation
One of the biggest challenges in troubleshooting today is gaining centralized visibility into application performance across metrics, infrastructure and logs. Without this unified visibility, it remains difficult to identify and resolve issues promptly. To address this challenge, organizations can leverage observability tools that provide a centralized overview of application performance and health.
By automatically discovering and inventorying all services running in the environment, these capabilities enable quick identification of performance metrics, errors, and infrastructure details related to each service. This comprehensive view allows teams to pinpoint the root cause of issues and accelerate the troubleshooting process.
Proactively monitoring and investigating application performance through AI-powered solutions and insights further enables organizations to identify and address potential problems before they impact end-users, reducing MTTR and improving overall system performance.
Tip 5
Infrastructure Performance Investigation
As organizations adopt distributed services and ephemeral infrastructure, troubleshooting becomes more complex. Managing Kubernetes environments, in particular, poses challenges due to their dynamic and ever-changing nature.
To streamline troubleshooting in Kubernetes, organizations need unified observability capabilities. These capabilities provide deep insights into infrastructure performance metrics, allowing teams to filter related data by clusters, namespaces, and deployments.
Additionally, organizations can investigate logs associated with specific services or pods, gaining valuable context for troubleshooting by using solutions that offer AI-backed analysis. By having a holistic view of the Kubernetes infrastructure, teams can identify performance bottlenecks, detect anomalies, and resolve issues swiftly.
This proactive approach to infrastructure performance investigation minimizes MTTR by reducing the time spent on identifying and troubleshooting issues in dynamic environments.
Discover How Logz.io Can Help Reduce MTTR
Reducing MTTR is crucial for maintaining optimal performance and user satisfaction. By implementing the five tips discussed here, organizations can streamline their troubleshooting processes and minimize downtime.
At Logz.io, users now have a ‘smart’ AI agent that can reason independently, helping them do a better job by extending their own technical capabilities. We’ve introduced the Logz.io AI Agent, which helps generate the analysis and action/response needed. Immediate benefits of this approach include reduced mean time to response (MTTR), increased confidence in new deployments, and accelerated software velocity—all critical results for an observability platform.
We’re seeing the agent evolve and improve every day—however, strong results have been evident since we first introduced it to customers.
The AI Agent provides Logz.io customers with these critical capabilities:
AI Agent for Data Analysis: Through an intuitive, chat-based interface, users interact with their data in real time, posing complex questions in plain language, and receiving insights without manual querying or navigating multiple dashboards.
AI Agent for Root Cause Analysis (RCA): Via automated investigation, the AI Agent diagnoses the root causes of system issues, delivering detailed insights and actionable recommendations to dramatically reduce troubleshooting timeframes.
Employ natural language interaction and analyze available telemetry data to gain a detailed understanding of system status and health. Moving directly from issue detection to automated investigation, you’ll dramatically simplify and reduce the time from discovery to response.
Customers who have used our AI Agent during beta availability have realized the following benefits:
70% reduction
in manual troubleshooting, streamlining operational workflows and empowering teams to focus on innovation.
5x faster
root cause analysis, enabling teams to quickly diagnose and address issues without extensive manual intervention.
3x faster
system recovery, minimizing downtime and ensuring reliable system performance.
See how these capabilities can reduce your MTTR with a demo of Logz.io. Sign up here.
Get started for free
Completely free for 14 days, no strings attached.