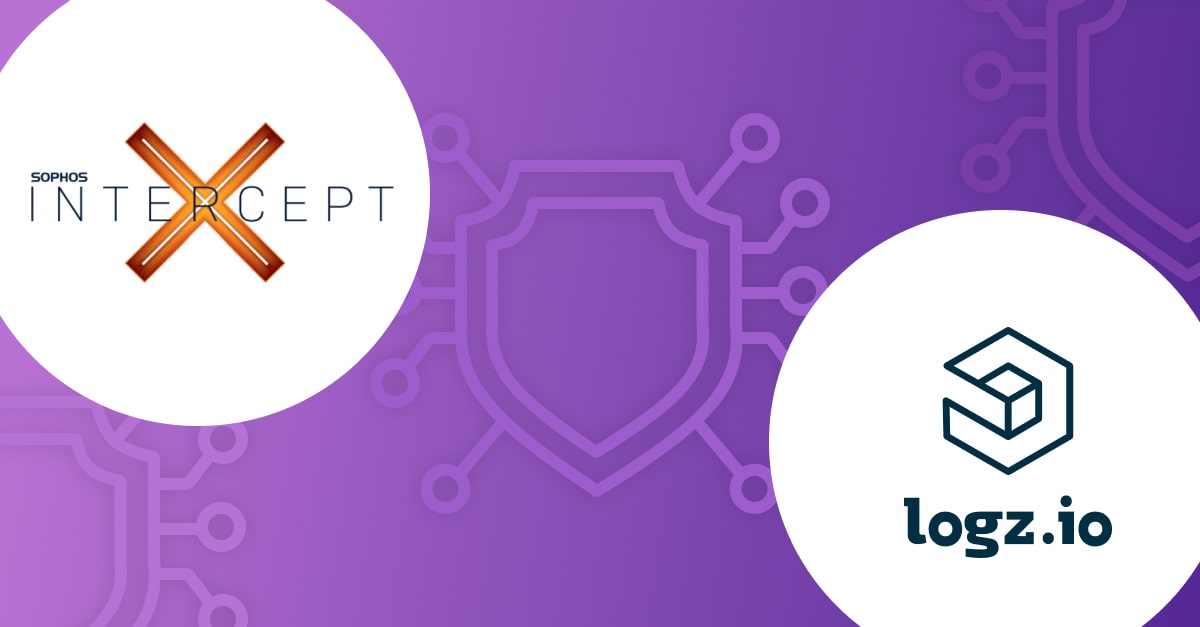
Building AIOps Now for the Future
AIOps is a term Gartner invented to describe a general trend of applying AI techniques to IT Operations data sources to provide additional insights and scale to the teams operating today’s complex software system. AIOps is essentially a feature or set of features to analyze, combine, and collect data. Unfortunately, the lack of AI in these solutions often turns many people off, but this promise is still possible.
What is AIOps?
The Gartner definition defines that “AIOps platforms” are platforms that “utilize big data, modern machine learning and other advanced analytics technologies to directly and indirectly enhance IT operations (monitoring, automation and service desk) functions with proactive, personal and dynamic insight.” The definition continues that AIOps platforms also “enable the concurrent use of multiple data sources, data collection methods, analytical (real-time and deep) technologies, and presentation technologies.”
Much of today’s AIOps platforms are relegated as expert systems that machine learning and other mathematics underpin. There is a lot of AIOps bashing going on out there, but a general lack of understanding that these technologies do in fact provide tangible benefits seems to be ignored. Part of the challenge is that there are no true AIOps products (contrary to what vendors may say). However, there are products which provide benefits to users, using AIOps capabilities.
Domain-Agnostic vs. Domain-Centric AIOps
Gartner describes two distinct types of AIOps products those which are domain-agnostic and those which are domain-centric.
Examples of this are the difference between a generic data aggregation tool (observability platforms, event correlation tools, and even some time-series analysis tools) versus domain-specific tools such as service management platforms and monitoring tools which target applications, networks, or general infrastructure.
There is a market guide that Gartner publishes, but there is no current plan for a magic quadrant yet. Sometimes, market guides move into a magic quadrant, but often they do not. Gartner publishes 3-4 times as many market guides as magic quadrants because of the maturity and depth of the magic quadrant research versus the market guide. The reason AIOps and other research areas aren’t a magic quadrant is that the market has not yet formed and these solutions are very diverse in their use cases and user types.
Gartner suggests users focus on the most pragmatic use cases and outcomes regarding AIOps given the lack of maturity. Focus on what the tools can do to meet immediate needs versus what AIOps cannot deliver yet. Today, many of these AIOps capabilities can really help teams scale and provide closed loop automation.
In APM tools, we’ve seen those which help with problem isolation. We look at the metrics along the graph of relationships between components on a transaction trace to determine a root cause. This is available in most leading commercial APM tools today and is proliferating to other types of observability.
Similarly, the use of AIOps capabilities in ITSM can speed up or automate ticket remediation, which is why we’ve seen advancement and acquisitions in this space.
Challenges
The challenges are that much of the current AIOps tooling uses unsupervised machine learning on this data to create baselines, clustering, and other aggregations to build anomaly detection. Anomaly detection doesn’t work very well, as the anomalies happen often enough that it creates toil for engineering teams. There are examples of supervised learning which works more effectively to find actual issues and surfacing those.
The reason Gartner includes CI/CD systems in AIOps because many of those platforms create closed-loop systems which take observability data to determine if a deployment in canary is going the right way and should be rolled out. These systems do so automatically, without user intervention.
There are technologies and tools such as ours at Logz.io doing supervised machine learning on crowdsourced data along with anomaly detection providing useful, actionable alerts which we can act upon. AIOps technologies can be very useful to teams, as our customers can attest to, but there are still false positives and false negatives.
The Future of AIOps
AIOps still has some distance to cover in order to mature.
We have plans for the next generation of supervised capabilities in our platform, and going down that path is the most benefit to users, along with leveraging cloud services from Google, Microsoft, Amazon, and IBM, etc. There is also much promise with open source machine learning projects such as TensorFlow, Knime, Scikit-learn and others.
AIOps is helping today, but it needs more work.
Get started for free
Completely free for 14 days, no strings attached.