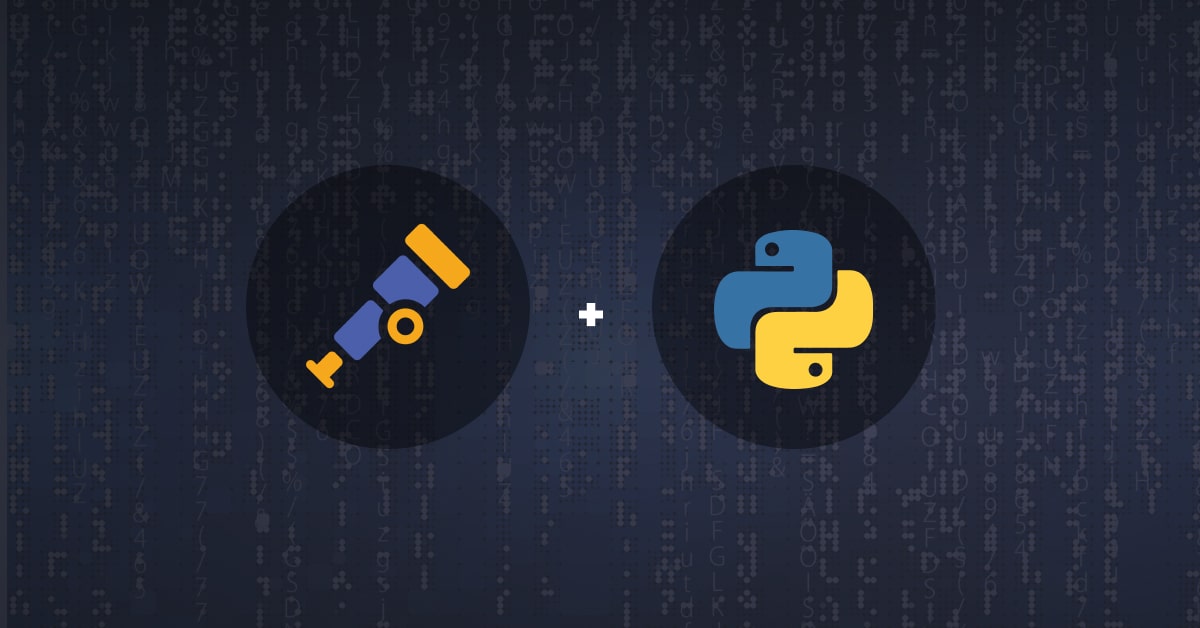
The Evolution of Open Source Observability
On May 27, the first OpenObservability Conference was held to bring together leaders, practitioners, and users of leading open source observability tools for sessions on the experiences, strategies, and future of the industry. For the Logz.io team, as long-time proponents of open source, it was rewarding to see everyone come together to explore the challenges and opportunities of open source observability. At the same time, it was great to hear from experts about practical tips and best practices engineers are implementing around the globe.
While our team will be building on the momentum of OpenObservability with upcoming projects and events for the community, we wanted to highlight some of the sessions from this first event and share some of the key takeaways.
Up first, was the session led by Logz.io CEO, Tomer Levy, who shared his view on the future of open source observability. Tomer walked attendees through the history of application monitoring, namely the current state and challenges presented by distributed applications and microservices.. He also made plain the opportunities ahead for engineers to draw on machine learning to ensure more performant and dynamic cloud applications or infrastructure.
Monitoring Monolith Applications
In the beginning, engineers and developers worked on monolith applications. Monitoring monolith applications were much less complex since each engineer would monitor only for specific use cases and use a specialized tool for the use case he or she worked on.
In addition, engineers worked with static infrastructure and used proprietary tools for monitoring. While this process was far less complex, it didn’t come without major challenges.
For example, collecting data was extremely difficult, so eventually organizations began to move away from this technology as more flexible options began to emerge.
The Move toward Monitoring Distributed Applications
Next, the industry moved to virtual machines which enabled engineering teams to monitor multiple VMs on the same server. But as a result of this move, engineers were responsible for performance, which led to the evolution of APMs (Application Performance Management).
During this time, new tools and technologies emerged to make monitoring performance easier, but many of the tools were proprietary, forcing engineers to be “locked” in by the vendor and unable to modify the software or easily transition between different solutions.
Unfortunately, this has also resulted in less interoperable systems and an inability to look at all the data holistically, from the data center to all the applications.
The Cloud Era
With the move to the cloud, all of our data is stored in one place, a more holistic option for Ops. There has also been an explosion of of open source observability tools to monitor application performance.
Many organizations, as part of their DevOps and cloud environment processes, also began creating an observability stack comprised of logs, metrics, and traces to increase visibility into their systems. With the help of numerous open source based observability offerings, many problems were solved….but other challenges persisted.
Despite the emergence of the cloud, there are still many gaps we need to close to become better at gaining observability. Teams are running and having to scale more and more observability tools to keep pace with new cloud services in development, As a result, engineers have to know networking, application layers, and containers—especially container orchestration via Kubernetes.
Clearly, we have evolved from an era where Ops roles were essentially SysAdmins tasked with relatively reactive monitoring and maintenance. Nowadays, we live and work in a reality where these engineers are comparable to data scientists and must have deep analytical skills to get the right insights from their systems.
The Promise of Machine Learning
With more data consisting of logs, metrics, and traces, data-centric DevOps engineers can unify this information together in one place to easily teach machines to analyze this data, become smarter, and get the full context of an event.
But for machine learning to be effective you need to use structured data. And logs, metrics, and traces tend to be fragmented.
That’s why projects like OpenTelemetry and others like it are extremely critical for powering these advancements. The more structure we create for these data sets, the more we escalate innovation so that machine learning technologies can easily look at data systematically to produce insights.
Today, we have data, we have structure, and we have widespread access to machine learning, powered by many of the leading cloud providers.
This facilitates even smaller companies’ access to machine learning’s transformative capabilities and engineering teams’ extraction of insights. As a result, those insights improve observability through both supervised and unsupervised machine learning.
With supervised learning, we can use human talent to teach machines how to effectively analyze structured data. Hence, supervised machine learning has many advantages over unsupervised machine learning
The Future of Open Source Observability
In the next few years, we’ll see a new generation of observability technologies. They will comprise early detection, automatic troubleshooting, and anomaly detection looking at data with unprecedented accuracy. This means our DevOps teams won’t be jumping over every production issue. Instead, they will be in charge of teaching and classifying the machines to automatically detect issues on their own.
After all, if taught properly, machines have the capability of breaking through the noise and effectively finding insights much faster than humans. As a result, engineers will be able to focus on larger events, while machines take care of mundane tasks. This technology will not only be available for large organizations, but to startups and small businesses as well.
It’s a very exciting time. If you take a look at how we have evolved from monolith applications and proprietary software solutions, to where we are today, on the verge of major breakthroughs with machine learning. In addition, there’s a lot of room for open source projects to apply these tools to standard modeling and data sets.
The more we use these structured data sets, the better place we’ll be in to use machine learning and open source tools to facilitate engineers’ jobs make their work more impactful.
To see the full talk, check out the video below!
Get started for free
Completely free for 14 days, no strings attached.